Dell PowerEdge R940xa GPU Database Acceleration on - Page 16
NY TAXI Benchmarking on PowerEdge R940xa with Brytlyt
![]() |
View all Dell PowerEdge R940xa manuals
Add to My Manuals
Save this manual to your list of manuals |
Page 16 highlights
5 Brytlyt + Dell EMC PowerEdge R940xa Benchmarking Extensive benchmarking was done on pre-release Dell R940xa hardware and the results were impressive. 5.1 TPC-H benchmarking on PowerEdge R940xa with Brytlyt Using TPC-H data and queries, a single Dell 940xa Server with four NVIDIA P100 GPUs was able to achieve through-put of 1.9 billion rows per second at 223 GB/second of raw data for Query 1 and 16.8 billion rows per second at 1.8 TB/second of raw data for Query 6. Join performance was also very good, processing 121 million rows per second when joining the three largest tables (line item 372 million rows, orders 93 million rows, customer 9 million rows). 5.2 NY TAXI Benchmarking on PowerEdge R940xa with Brytlyt The NY Taxi dataset is made up of 1.1 billion taxi trips conducted in New York City between 2009 and 2015. In CSV format the data is about 500 GB in size. Benchmarking using the NY Taxi data was just as impressive and there were significant improvements when compared to earlier Brytlyt benchmarking. This was particularly evident for the more complex queries. Because only a single machine with four P100 GPUs was used, the original dataset had to be scaled down from 1.1 billion rows to 243 million rows. Absolute run times were better but as only one machine was used, the overhead of coordinating multiple machines over the network is not included in the runtime. 5.3 PowerEdge R940xa NY Taxi data runtimes Row Count 243,771,304 Query Q1 Q2 Q3 Q4 Run Time (ms) 3 16 99 146 5.4 Previous Benchmark Row Count Query Run Time (ms) 1,100,000,000 Q1 Q2 5 11 Q3 Q4 103 188 5.5 Reducing Time-to-Value for Data Analysts This paradigm shift in query performance can massively reduce the time it takes Data Scientists and Analysts to perform queries. For a business this means: Giving business leaders better situational awareness on large amounts of high velocity data Empowering data scientists to interactively explore large datasets for detailed insights Helping data analysts answer crucial business questions in real time. 16 GPU Database Acceleration on PowerEdge R940xa
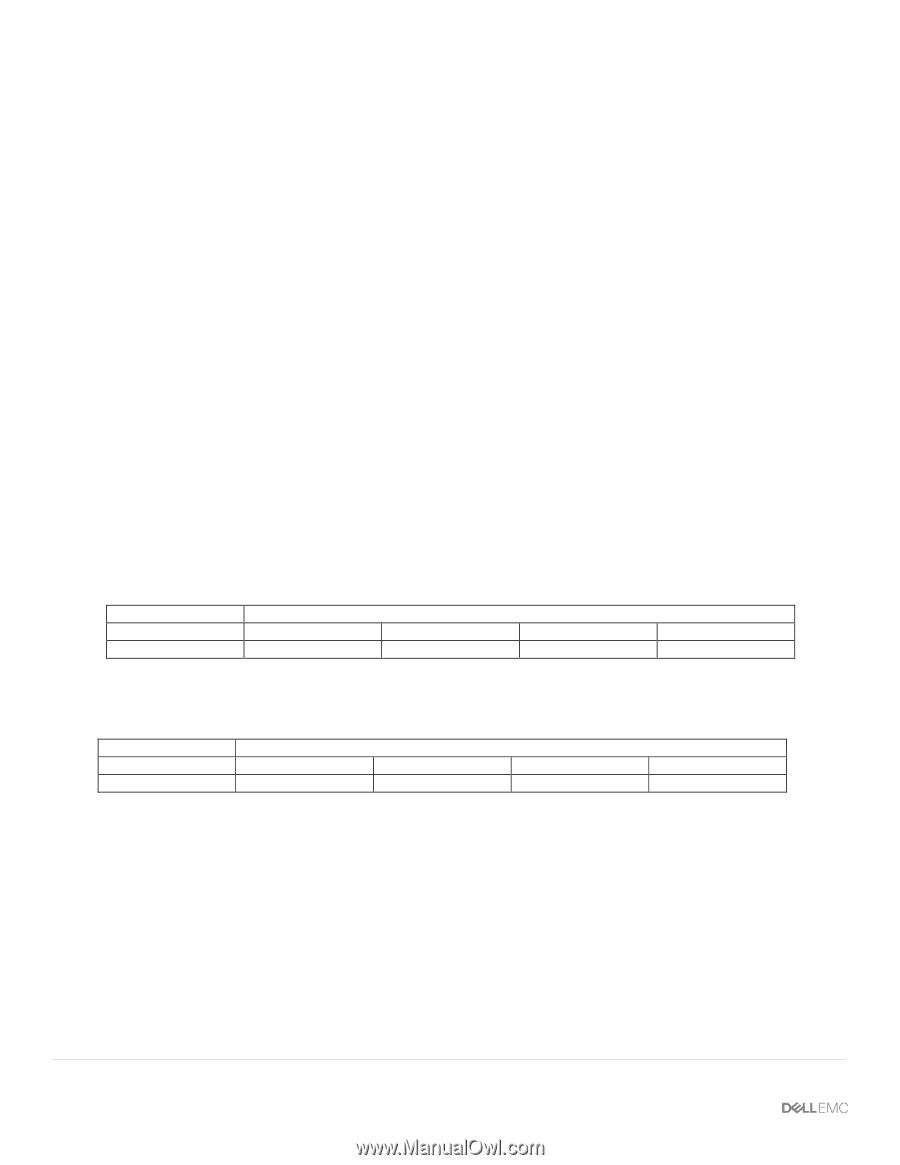